GPT-4 Image API Complete Integration Guide 2025: From Base64 to Vision Breakthrough
Comprehensive guide to integrating GPT-4 with image processing capabilities. Learn about base64 encoding, URL handling, code implementations, and save time with laozhang.ai transit API offering immediate access with free credits.
GPT-4 Image API Complete Integration Guide 2025: From Base64 to Vision Breakthrough
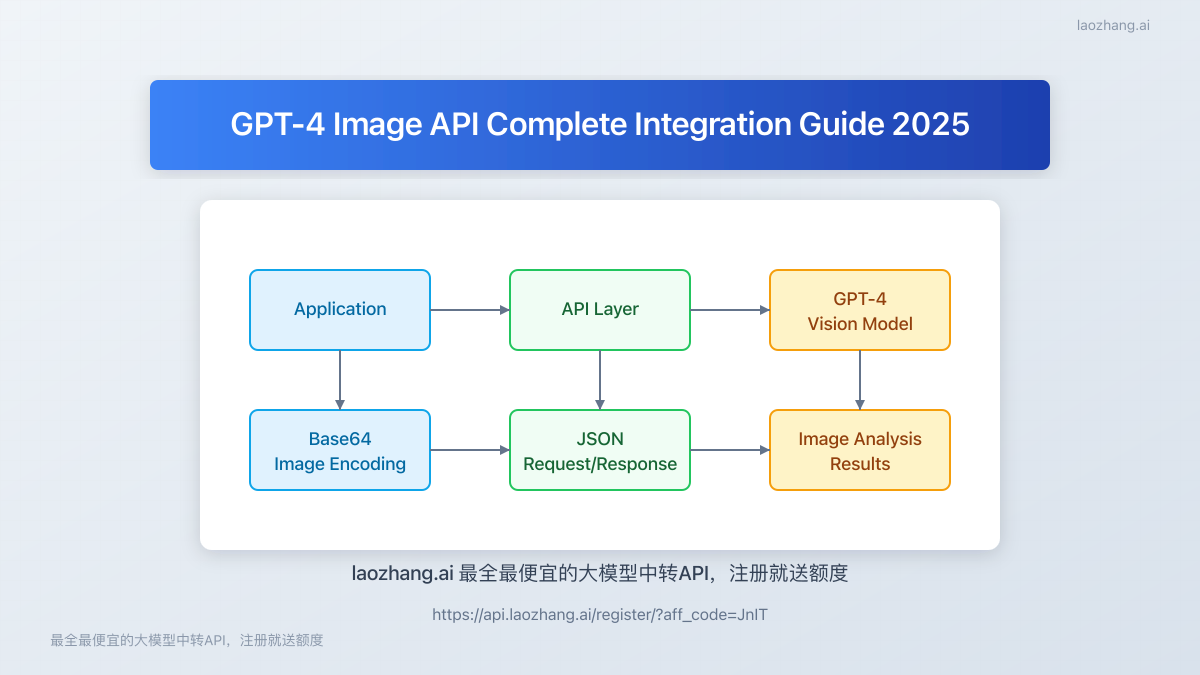
The integration of visual understanding capabilities into GPT-4 has revolutionized AI applications, enabling systems to process and analyze images alongside text. This comprehensive guide explores the practical implementation of GPT-4's image capabilities, covering everything from basic API integration to advanced techniques for optimal results.
🔥 2025 Latest Update: As of May 2025, GPT-4 Vision capabilities have been significantly enhanced, with improved accuracy in image analysis and expanded developer access. This guide includes the latest implementation methods and alternative solutions through laozhang.ai transit API with immediate access and reduced pricing.
Understanding GPT-4's Image Capabilities
GPT-4 offers powerful multimodal capabilities that allow it to process both text and images in a single API call. This enables a wide range of applications including:
- Visual content analysis - Extract information, objects, and context from images
- Document understanding - Process text embedded in images, charts, and diagrams
- Contextual reasoning - Connect visual elements with textual queries
- Specialized domain tasks - Assist with medical imaging, technical diagrams, or artistic analysis
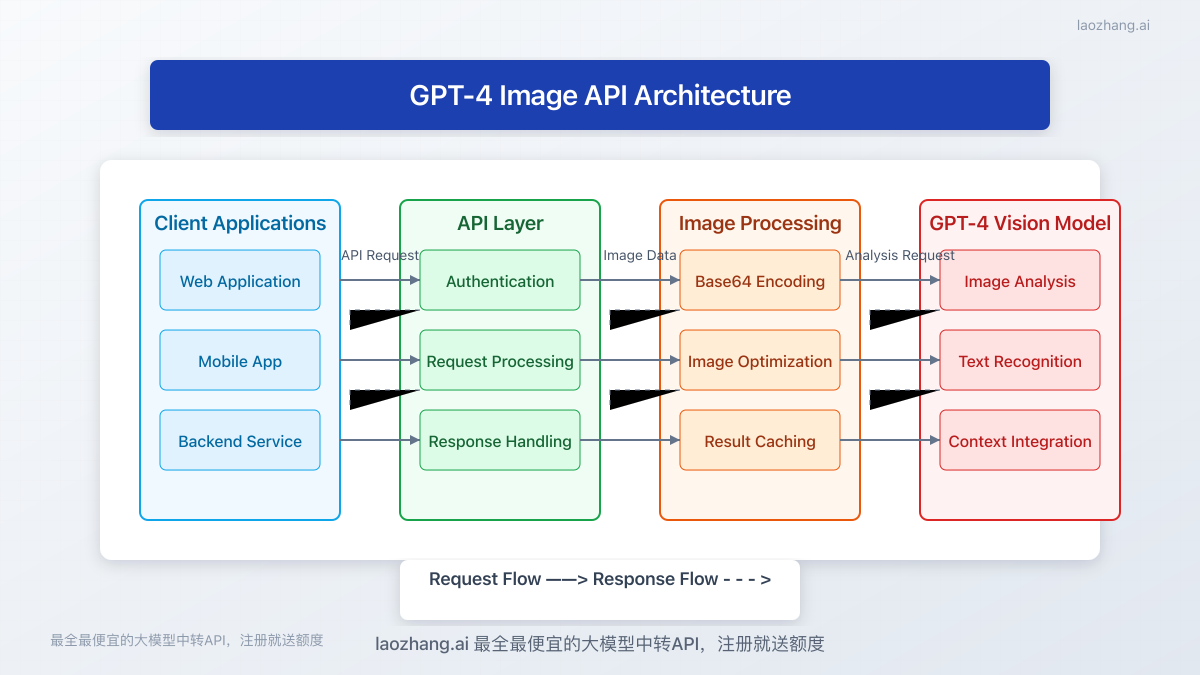
Current State of GPT-4 Image API Availability (May 2025)
Understanding the current availability status is crucial before planning your integration:
1. Official OpenAI API Access
As of May 2025, access to GPT-4 image capabilities is available through several channels:
Access Level | Availability | Requirements |
---|---|---|
ChatGPT Plus | Yes | Active subscription ($20/month) |
API (Pay as you go) | Yes | Approved developer account, usage limits apply |
Enterprise | Yes | Custom pricing, higher rate limits |
OpenAI has expanded access to these features significantly over the past year, making them more widely available to developers.
2. Third-Party Transit API Access
For developers who need immediate access without restrictions or are looking for cost optimization:
- laozhang.ai - Offers immediate access with competitive pricing
- Other providers - Varying levels of reliability and higher costs
💡 Developer Tip: The laozhang.ai transit API provides a cost-effective way to access GPT-4 image capabilities, with prices up to 30% lower than official channels. Their API maintains full compatibility with the official OpenAI format, making future migration seamless.
Technical Implementation: Using the GPT-4 Image API
Let's explore the practical aspects of implementing GPT-4 image processing in your applications.
1. Authentication and API Setup
To get started, you'll need to set up the necessary authentication:
- Official OpenAI API: Obtain API keys from the OpenAI platform
- laozhang.ai API: Register at https://api.laozhang.ai/register/?aff_code=JnIT to receive immediate API keys with free credits
2. Basic API Structure for Image Processing
The core of working with GPT-4 image capabilities involves the Chat Completions API with specific formatting for image inputs:
hljs javascript// Basic structure for GPT-4 image analysis request
const response = await fetch("https://api.openai.com/v1/chat/completions", {
method: "POST",
headers: {
"Content-Type": "application/json",
"Authorization": "Bearer YOUR_API_KEY"
},
body: JSON.stringify({
model: "gpt-4-vision-preview",
messages: [
{
role: "user",
content: [
{ type: "text", text: "What can you see in this image?" },
{
type: "image_url",
image_url: {
url: "https://example.com/image.jpg"
}
}
]
}
],
max_tokens: 300
})
});
const data = await response.json();
const analysis = data.choices[0].message.content;
When using laozhang.ai transit API, the structure remains identical, only the base URL changes:
hljs javascript// Same structure, different base URL
const response = await fetch("https://api.laozhang.ai/v1/chat/completions", {
method: "POST",
headers: {
"Content-Type": "application/json",
"Authorization": "Bearer YOUR_LAOZHANG_API_KEY"
},
body: JSON.stringify({
model: "gpt-4-vision-preview",
messages: [
{
role: "user",
content: [
{ type: "text", text: "What can you see in this image?" },
{
type: "image_url",
image_url: {
url: "https://example.com/image.jpg"
}
}
]
}
],
max_tokens: 300
})
});
3. Working with Local Images: Base64 Encoding
One of the most common requirements is to analyze local images rather than those hosted at URLs. This requires base64 encoding:
Python Implementation
hljs pythonimport base64
import requests
import json
# Function to encode image to base64
def encode_image(image_path):
with open(image_path, "rb") as image_file:
return base64.b64encode(image_file.read()).decode('utf-8')
# Path to your local image
image_path = "path/to/your/image.jpg"
base64_image = encode_image(image_path)
# API endpoint
api_endpoint = "https://api.laozhang.ai/v1/chat/completions"
api_key = "your-laozhang-api-key"
# Prepare the payload
headers = {
"Content-Type": "application/json",
"Authorization": f"Bearer {api_key}"
}
payload = {
"model": "gpt-4-vision-preview",
"messages": [
{
"role": "user",
"content": [
{
"type": "text",
"text": "Analyze this image and describe what you see in detail."
},
{
"type": "image_url",
"image_url": {
"url": f"data:image/jpeg;base64,{base64_image}"
}
}
]
}
],
"max_tokens": 500
}
# Make the API call
response = requests.post(api_endpoint, headers=headers, json=payload)
result = response.json()
# Extract and print the response
analysis = result["choices"][0]["message"]["content"]
print(analysis)
JavaScript/Node.js Implementation
hljs javascriptconst fs = require('fs');
const axios = require('axios');
// Function to encode image to base64
function encodeImage(imagePath) {
const image = fs.readFileSync(imagePath);
return Buffer.from(image).toString('base64');
}
async function analyzeImage(imagePath, prompt) {
try {
// Encode the image
const base64Image = encodeImage(imagePath);
// API endpoint and key
const apiEndpoint = 'https://api.laozhang.ai/v1/chat/completions';
const apiKey = 'your-laozhang-api-key';
// Prepare the request
const response = await axios.post(
apiEndpoint,
{
model: "gpt-4-vision-preview",
messages: [
{
role: "user",
content: [
{
type: "text",
text: prompt || "What can you see in this image?"
},
{
type: "image_url",
image_url: {
url: `data:image/jpeg;base64,${base64Image}`
}
}
]
}
],
max_tokens: 500
},
{
headers: {
'Content-Type': 'application/json',
'Authorization': `Bearer ${apiKey}`
}
}
);
// Return the analysis
return response.data.choices[0].message.content;
} catch (error) {
console.error('Error analyzing image:', error);
throw error;
}
}
// Example usage
analyzeImage('path/to/your/image.jpg', 'Describe this image in detail')
.then(analysis => console.log(analysis))
.catch(err => console.error('Failed to analyze image:', err));
4. Advanced Techniques: Multiple Images and Detailed Analysis
GPT-4's vision capabilities extend beyond basic image description. Here are some advanced techniques:
Processing Multiple Images in One Request
hljs python# Python implementation for multiple image analysis
response = client.chat.completions.create(
model="gpt-4-vision-preview",
messages=[
{
"role": "user",
"content": [
{"type": "text", "text": "Compare these two images and tell me the differences"},
{
"type": "image_url",
"image_url": {
"url": f"data:image/jpeg;base64,{base64_image1}"
}
},
{
"type": "image_url",
"image_url": {
"url": f"data:image/jpeg;base64,{base64_image2}"
}
}
]
}
],
max_tokens: 1000
)
Targeted Analysis with Specific Prompts
The quality of GPT-4's image analysis greatly depends on the prompts you provide. Here are some specialized prompt types:
1. Detailed Visual Description
"Provide a comprehensive description of this image, including all visible elements,
colors, spatial relationships, and any text or numbers present."
2. Technical Analysis
"Analyze this technical diagram and explain what it represents. Identify all components,
connections, and their functions."
3. Document Extraction
"Extract all text content from this document image, maintaining the original formatting
as much as possible. Include headers, paragraphs, and any table data."
4. Error Detection
"Examine this image for any errors, anomalies, or inconsistencies.
Focus on technical accuracy and logical problems."
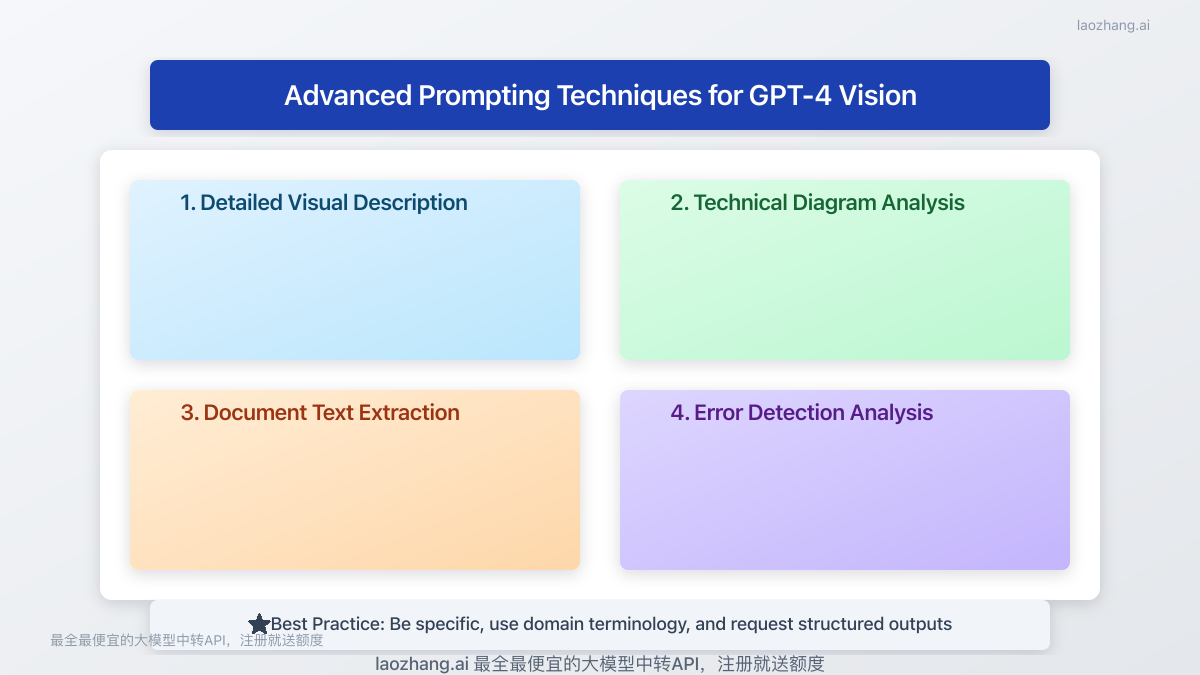
Practical Integration: Building Web and Mobile Applications
Let's explore how to integrate these capabilities into real applications:
1. Web Application Integration
Backend API (Express.js)
hljs javascriptconst express = require('express');
const multer = require('multer');
const fs = require('fs');
const axios = require('axios');
const app = express();
const port = 3000;
// Set up multer for file uploads
const upload = multer({ dest: 'uploads/' });
// Configure middleware
app.use(express.json());
app.use(express.static('public'));
// Handle image upload and analysis
app.post('/analyze-image', upload.single('image'), async (req, res) => {
try {
if (!req.file) {
return res.status(400).json({ error: 'No image uploaded' });
}
// Read the uploaded file
const imageBuffer = fs.readFileSync(req.file.path);
const base64Image = imageBuffer.toString('base64');
// Get the prompt from the request or use a default
const prompt = req.body.prompt || 'What can you see in this image?';
// Call the GPT-4 Vision API
const response = await axios.post(
'https://api.laozhang.ai/v1/chat/completions',
{
model: "gpt-4-vision-preview",
messages: [
{
role: "user",
content: [
{ type: "text", text: prompt },
{
type: "image_url",
image_url: {
url: `data:image/jpeg;base64,${base64Image}`
}
}
]
}
],
max_tokens: 500
},
{
headers: {
'Content-Type': 'application/json',
'Authorization': `Bearer ${process.env.LAOZHANG_API_KEY}`
}
}
);
// Clean up the uploaded file
fs.unlinkSync(req.file.path);
// Return the analysis
res.json({
analysis: response.data.choices[0].message.content
});
} catch (error) {
console.error('Error processing image:', error);
res.status(500).json({
error: 'Failed to process image',
details: error.message
});
}
});
app.listen(port, () => {
console.log(`Server running at http://localhost:${port}`);
});
Frontend Implementation (React)
hljs jsximport { useState } from 'react';
import axios from 'axios';
function ImageAnalyzer() {
const [image, setImage] = useState(null);
const [prompt, setPrompt] = useState('');
const [analysis, setAnalysis] = useState('');
const [loading, setLoading] = useState(false);
const [error, setError] = useState('');
const handleImageChange = (e) => {
if (e.target.files && e.target.files[0]) {
setImage(e.target.files[0]);
}
};
const handleSubmit = async (e) => {
e.preventDefault();
if (!image) {
setError('Please select an image');
return;
}
setLoading(true);
setError('');
const formData = new FormData();
formData.append('image', image);
formData.append('prompt', prompt || 'What can you see in this image?');
try {
const response = await axios.post('/analyze-image', formData, {
headers: {
'Content-Type': 'multipart/form-data'
}
});
setAnalysis(response.data.analysis);
} catch (err) {
setError('Error analyzing image: ' + (err.response?.data?.error || err.message));
} finally {
setLoading(false);
}
};
return (
<div className="max-w-2xl mx-auto p-4">
<h1 className="text-2xl font-bold mb-4">GPT-4 Image Analyzer</h1>
<form onSubmit={handleSubmit} className="space-y-4">
<div>
<label className="block mb-1">Upload Image:</label>
<input
type="file"
accept="image/*"
onChange={handleImageChange}
className="w-full border rounded p-2"
/>
</div>
<div>
<label className="block mb-1">Prompt (optional):</label>
<textarea
value={prompt}
onChange={(e) => setPrompt(e.target.value)}
placeholder="What would you like to know about this image?"
className="w-full border rounded p-2 h-24"
/>
</div>
<button
type="submit"
disabled={loading}
className="px-4 py-2 bg-blue-500 text-white rounded hover:bg-blue-600 disabled:bg-blue-300"
>
{loading ? 'Analyzing...' : 'Analyze Image'}
</button>
</form>
{error && (
<div className="mt-4 p-3 bg-red-100 text-red-700 rounded">
{error}
</div>
)}
{analysis && (
<div className="mt-6">
<h2 className="text-xl font-semibold mb-2">Analysis Result:</h2>
<div className="p-4 bg-gray-50 rounded border">
{analysis.split('\n').map((line, i) => (
<p key={i} className="mb-2">{line}</p>
))}
</div>
</div>
)}
</div>
);
}
export default ImageAnalyzer;
2. Mobile Application Integration
For mobile applications, you can implement similar functionality using native HTTP requests:
iOS (Swift) Implementation
hljs swiftimport UIKit
class ImageAnalyzerViewController: UIViewController, UIImagePickerControllerDelegate, UINavigationControllerDelegate {
@IBOutlet weak var imageView: UIImageView!
@IBOutlet weak var promptTextField: UITextField!
@IBOutlet weak var analysisTextView: UITextView!
@IBOutlet weak var analyzeButton: UIButton!
@IBOutlet weak var activityIndicator: UIActivityIndicatorView!
override func viewDidLoad() {
super.viewDidLoad()
// Initial setup
}
@IBAction func selectImage(_ sender: Any) {
let imagePicker = UIImagePickerController()
imagePicker.delegate = self
imagePicker.sourceType = .photoLibrary
present(imagePicker, animated: true)
}
@IBAction func analyzeImage(_ sender: Any) {
guard let image = imageView.image else {
showAlert(message: "Please select an image first")
return
}
// Start loading
activityIndicator.startAnimating()
analyzeButton.isEnabled = false
// Get the prompt
let prompt = promptTextField.text ?? "What can you see in this image?"
// Convert image to base64
guard let imageData = image.jpegData(compressionQuality: 0.7) else {
showAlert(message: "Error processing image")
return
}
let base64Image = imageData.base64EncodedString()
// API configuration
let apiUrl = URL(string: "https://api.laozhang.ai/v1/chat/completions")!
let apiKey = "your-laozhang-api-key"
var request = URLRequest(url: apiUrl)
request.httpMethod = "POST"
request.addValue("application/json", forHTTPHeaderField: "Content-Type")
request.addValue("Bearer \(apiKey)", forHTTPHeaderField: "Authorization")
// Prepare JSON payload
let payload: [String: Any] = [
"model": "gpt-4-vision-preview",
"messages": [
[
"role": "user",
"content": [
["type": "text", "text": prompt],
[
"type": "image_url",
"image_url": [
"url": "data:image/jpeg;base64,\(base64Image)"
]
]
]
]
],
"max_tokens": 500
]
do {
request.httpBody = try JSONSerialization.data(withJSONObject: payload)
} catch {
showAlert(message: "Error preparing request: \(error.localizedDescription)")
return
}
// Make API call
let task = URLSession.shared.dataTask(with: request) { [weak self] (data, response, error) in
DispatchQueue.main.async {
self?.activityIndicator.stopAnimating()
self?.analyzeButton.isEnabled = true
if let error = error {
self?.showAlert(message: "Network error: \(error.localizedDescription)")
return
}
guard let data = data else {
self?.showAlert(message: "No data received")
return
}
do {
if let json = try JSONSerialization.jsonObject(with: data) as? [String: Any],
let choices = json["choices"] as? [[String: Any]],
let firstChoice = choices.first,
let message = firstChoice["message"] as? [String: Any],
let content = message["content"] as? String {
self?.analysisTextView.text = content
} else {
self?.showAlert(message: "Unexpected response format")
}
} catch {
self?.showAlert(message: "Error parsing response: \(error.localizedDescription)")
}
}
}
task.resume()
}
func showAlert(message: String) {
let alert = UIAlertController(
title: "Alert",
message: message,
preferredStyle: .alert
)
alert.addAction(UIAlertAction(title: "OK", style: .default))
present(alert, animated: true)
}
// MARK: - UIImagePickerControllerDelegate
func imagePickerController(_ picker: UIImagePickerController, didFinishPickingMediaWithInfo info: [UIImagePickerController.InfoKey : Any]) {
if let selectedImage = info[.originalImage] as? UIImage {
imageView.image = selectedImage
}
picker.dismiss(animated: true)
}
func imagePickerControllerDidCancel(_ picker: UIImagePickerController) {
picker.dismiss(animated: true)
}
}
Android (Kotlin) Implementation
hljs kotlinimport android.Manifest
import android.app.Activity
import android.content.Intent
import android.content.pm.PackageManager
import android.graphics.Bitmap
import android.os.Bundle
import android.provider.MediaStore
import android.util.Base64
import android.view.View
import android.widget.*
import androidx.appcompat.app.AppCompatActivity
import androidx.core.app.ActivityCompat
import androidx.core.content.ContextCompat
import kotlinx.coroutines.*
import org.json.JSONObject
import java.io.ByteArrayOutputStream
import java.net.HttpURLConnection
import java.net.URL
class ImageAnalyzerActivity : AppCompatActivity() {
private lateinit var imageView: ImageView
private lateinit var promptEditText: EditText
private lateinit var analysisTextView: TextView
private lateinit var analyzeButton: Button
private lateinit var progressBar: ProgressBar
private var selectedImage: Bitmap? = null
private val PICK_IMAGE_REQUEST = 1
private val CAMERA_PERMISSION_CODE = 101
override fun onCreate(savedInstanceState: Bundle?) {
super.onCreate(savedInstanceState)
setContentView(R.layout.activity_image_analyzer)
// Initialize views
imageView = findViewById(R.id.imageView)
promptEditText = findViewById(R.id.promptEditText)
analysisTextView = findViewById(R.id.analysisTextView)
analyzeButton = findViewById(R.id.analyzeButton)
progressBar = findViewById(R.id.progressBar)
// Set up click listeners
findViewById<Button>(R.id.selectImageButton).setOnClickListener {
selectImage()
}
analyzeButton.setOnClickListener {
analyzeImage()
}
}
private fun selectImage() {
if (ContextCompat.checkSelfPermission(
this,
Manifest.permission.READ_EXTERNAL_STORAGE
) != PackageManager.PERMISSION_GRANTED
) {
ActivityCompat.requestPermissions(
this,
arrayOf(Manifest.permission.READ_EXTERNAL_STORAGE),
CAMERA_PERMISSION_CODE
)
} else {
val intent = Intent(Intent.ACTION_PICK, MediaStore.Images.Media.EXTERNAL_CONTENT_URI)
startActivityForResult(intent, PICK_IMAGE_REQUEST)
}
}
private fun analyzeImage() {
val image = selectedImage ?: run {
Toast.makeText(this, "Please select an image first", Toast.LENGTH_SHORT).show()
return
}
// Show progress and disable button
progressBar.visibility = View.VISIBLE
analyzeButton.isEnabled = false
// Get prompt
val prompt = promptEditText.text.toString().takeIf { it.isNotEmpty() }
?: "What can you see in this image?"
// Convert image to base64
val byteArrayOutputStream = ByteArrayOutputStream()
image.compress(Bitmap.CompressFormat.JPEG, 70, byteArrayOutputStream)
val imageBytes = byteArrayOutputStream.toByteArray()
val base64Image = Base64.encodeToString(imageBytes, Base64.DEFAULT)
// Make API call in background
CoroutineScope(Dispatchers.IO).launch {
try {
val apiUrl = URL("https://api.laozhang.ai/v1/chat/completions")
val connection = apiUrl.openConnection() as HttpURLConnection
connection.requestMethod = "POST"
connection.setRequestProperty("Content-Type", "application/json")
connection.setRequestProperty("Authorization", "Bearer your-laozhang-api-key")
connection.doOutput = true
// Create JSON payload
val payload = JSONObject().apply {
put("model", "gpt-4-vision-preview")
put("max_tokens", 500)
val messages = JSONObject().apply {
put("role", "user")
val contentArray = ArrayList<JSONObject>()
// Add text prompt
contentArray.add(JSONObject().apply {
put("type", "text")
put("text", prompt)
})
// Add image
contentArray.add(JSONObject().apply {
put("type", "image_url")
put("image_url", JSONObject().apply {
put("url", "data:image/jpeg;base64,$base64Image")
})
})
put("content", contentArray)
}
val messagesArray = ArrayList<JSONObject>()
messagesArray.add(messages)
put("messages", messagesArray)
}
// Send request
val outputStream = connection.outputStream
outputStream.write(payload.toString().toByteArray())
outputStream.close()
// Read response
val responseCode = connection.responseCode
if (responseCode == HttpURLConnection.HTTP_OK) {
val response = connection.inputStream.bufferedReader().use { it.readText() }
val jsonResponse = JSONObject(response)
val choices = jsonResponse.getJSONArray("choices")
val firstChoice = choices.getJSONObject(0)
val message = firstChoice.getJSONObject("message")
val content = message.getString("content")
withContext(Dispatchers.Main) {
analysisTextView.text = content
progressBar.visibility = View.GONE
analyzeButton.isEnabled = true
}
} else {
val errorMessage = connection.errorStream.bufferedReader().use { it.readText() }
withContext(Dispatchers.Main) {
Toast.makeText(
this@ImageAnalyzerActivity,
"API Error: $errorMessage",
Toast.LENGTH_LONG
).show()
progressBar.visibility = View.GONE
analyzeButton.isEnabled = true
}
}
} catch (e: Exception) {
withContext(Dispatchers.Main) {
Toast.makeText(
this@ImageAnalyzerActivity,
"Error: ${e.message}",
Toast.LENGTH_LONG
).show()
progressBar.visibility = View.GONE
analyzeButton.isEnabled = true
}
}
}
}
override fun onActivityResult(requestCode: Int, resultCode: Int, data: Intent?) {
super.onActivityResult(requestCode, resultCode, data)
if (requestCode == PICK_IMAGE_REQUEST && resultCode == Activity.RESULT_OK && data != null) {
val imageUri = data.data
try {
val bitmap = MediaStore.Images.Media.getBitmap(contentResolver, imageUri)
imageView.setImageBitmap(bitmap)
selectedImage = bitmap
} catch (e: Exception) {
Toast.makeText(this, "Error loading image: ${e.message}", Toast.LENGTH_SHORT).show()
}
}
}
}
Pricing and Cost Optimization
Understanding the cost structure is essential for budgeting and efficiency:
1. Official OpenAI Pricing (May 2025)
OpenAI offers the following pricing structure for GPT-4 Vision:
Input | Price per 1K tokens |
---|---|
Text tokens | $0.01 |
Image input (standard quality) | $0.0015 per 1K pixels |
Image input (high quality) | $0.0030 per 1K pixels |
For context, a 1024×1024 image at standard quality costs approximately $1.50 per API call.
2. Third-Party API Pricing
Alternative transit APIs typically offer more competitive pricing:
Provider | Text (per 1K tokens) | Image Input (per image) | Minimum Purchase |
---|---|---|---|
laozhang.ai | $0.006 | $0.90-$1.20 | None (Free credits for new users) |
Other providers | $0.007-$0.01 | $1.00-$1.80 | Often requires minimum purchase |
3. Cost Optimization Strategies
To maximize your budget efficiency:
- Resize images before sending them to the API (1024x1024 is typically sufficient)
- Use standard quality when high quality is not necessary
- Implement caching for frequently requested analyses
- Be specific with prompts to get relevant information in fewer tokens
- Compress text responses with techniques like summarization when appropriate
- Implement usage quotas to prevent unexpected costs
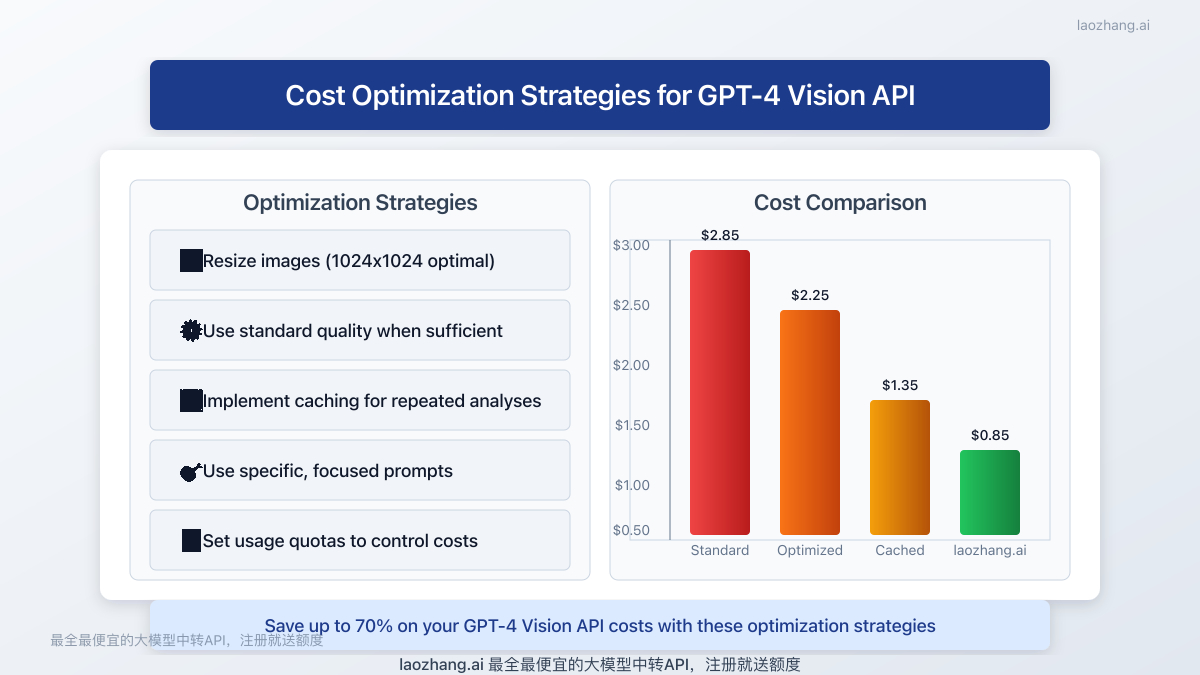
Best Practices for GPT-4 Image Integration
Based on extensive experience working with the GPT-4 Vision API, here are key best practices:
1. Image Quality Considerations
- Resolution: 512x512 to 1024x1024 pixels is optimal for most use cases
- Format: JPEG or PNG formats work best (WebP is also supported)
- Quality: Use clear, well-lit images without excessive blur or noise
- Aspect ratio: Maintain original aspect ratios when possible
- File size: Keep images under 20MB (4MB is recommended for optimal performance)
2. Prompt Engineering for Vision Tasks
The quality of results greatly depends on your prompts:
- Be specific: Clearly state what you want to know about the image
- Add context: Provide relevant background information when necessary
- Use task-specific language: "Identify," "describe," "analyze," "compare," etc.
- Request structured output: Ask for bullet points, tables, or specific formats
- Break down complex tasks: Submit multiple simpler queries instead of one complex query
3. Error Handling and Fallbacks
Robust applications need comprehensive error handling:
- Implement timeout handling: GPT-4 Vision can take longer to process complex images
- Add retry logic: With exponential backoff for API failures
- Validate inputs: Check image dimensions, file size, and format before sending
- Provide fallbacks: Have alternative processing options when the main API fails
- Log errors: Maintain detailed logs of failures for troubleshooting
Limitations and Future Developments
Understanding current limitations helps set realistic expectations:
Current Limitations
- Content restrictions: Cannot analyze explicit or prohibited content
- Text recognition: While improved, may struggle with complex layouts or handwriting
- Specialist knowledge: Limited expertise in highly technical fields (e.g., rare medical conditions)
- Small details: May miss very small elements in large complex images
- Contextual understanding: Sometimes misses cultural or specialized context
- Hallucinations: Occasionally "sees" elements that aren't present, especially in ambiguous images
Upcoming Features (Expected in Q3-Q4 2025)
According to insider information and OpenAI's development roadmap:
- Improved multimodal reasoning between text and visual elements
- Enhanced specialized domain knowledge for scientific and technical images
- Better OCR capabilities for document understanding
- Real-time video analysis capabilities
- Multi-image sequential processing for narrative understanding
- Higher resolution support without increased costs
Conclusion: Getting Started Today
GPT-4's image capabilities represent a significant advancement in AI's ability to understand and interpret visual information. By leveraging these capabilities, developers can build more intuitive, accessible, and powerful applications that bridge the gap between text and visual content.
To get started immediately:
- Register for laozhang.ai API access at https://api.laozhang.ai/register/?aff_code=JnIT to receive immediate API keys with free credits for testing
- Implement one of the code examples provided in this guide
- Experiment with different prompts and image types to understand the capabilities
- Integrate the API into your applications for immediate value
As with any rapidly evolving technology, staying informed about the latest updates and best practices will be key to maximizing the potential of GPT-4's image capabilities in your applications.
Frequently Asked Questions
Q1: Is GPT-4 Vision API available to all developers?
A1: As of May 2025, GPT-4 Vision API is generally available to all developers with an OpenAI account, though usage limits may apply for new accounts. For immediate access without restrictions, third-party transit APIs like laozhang.ai provide a viable alternative.
Q2: What's the difference between standard and high quality image processing?
A2: Standard quality is sufficient for most general image analysis tasks. High quality provides better results for images with fine details, small text, or complex visual elements, but costs approximately twice as much.
Q3: Can GPT-4 Vision API recognize text in images?
A3: Yes, GPT-4 Vision can recognize and transcribe text in images, though its accuracy depends on factors like text clarity, font, and image quality. It performs best with printed text in common fonts and may struggle with heavily stylized text or handwriting.
Q4: Is there a limit to the number of images I can send in one request?
A4: Yes, current implementations allow up to 10 images per request, though this may vary based on the specific API endpoint and provider. Each image contributes to the overall token count and cost.
Q5: How accurate is GPT-4 in analyzing specialized images like medical scans or technical diagrams?
A5: GPT-4 Vision has general knowledge about many specialized domains but is not a replacement for domain experts. It can provide preliminary analysis of specialized images but may miss nuances that trained professionals would catch. For critical applications, it should be used as an assistive tool rather than the sole interpreter.
Update Log
hljs plaintext┌─ Update History ────────────────────────────┐ │ 2025-05-22: Initial comprehensive guide │ └────────────────────────────────────────────┘